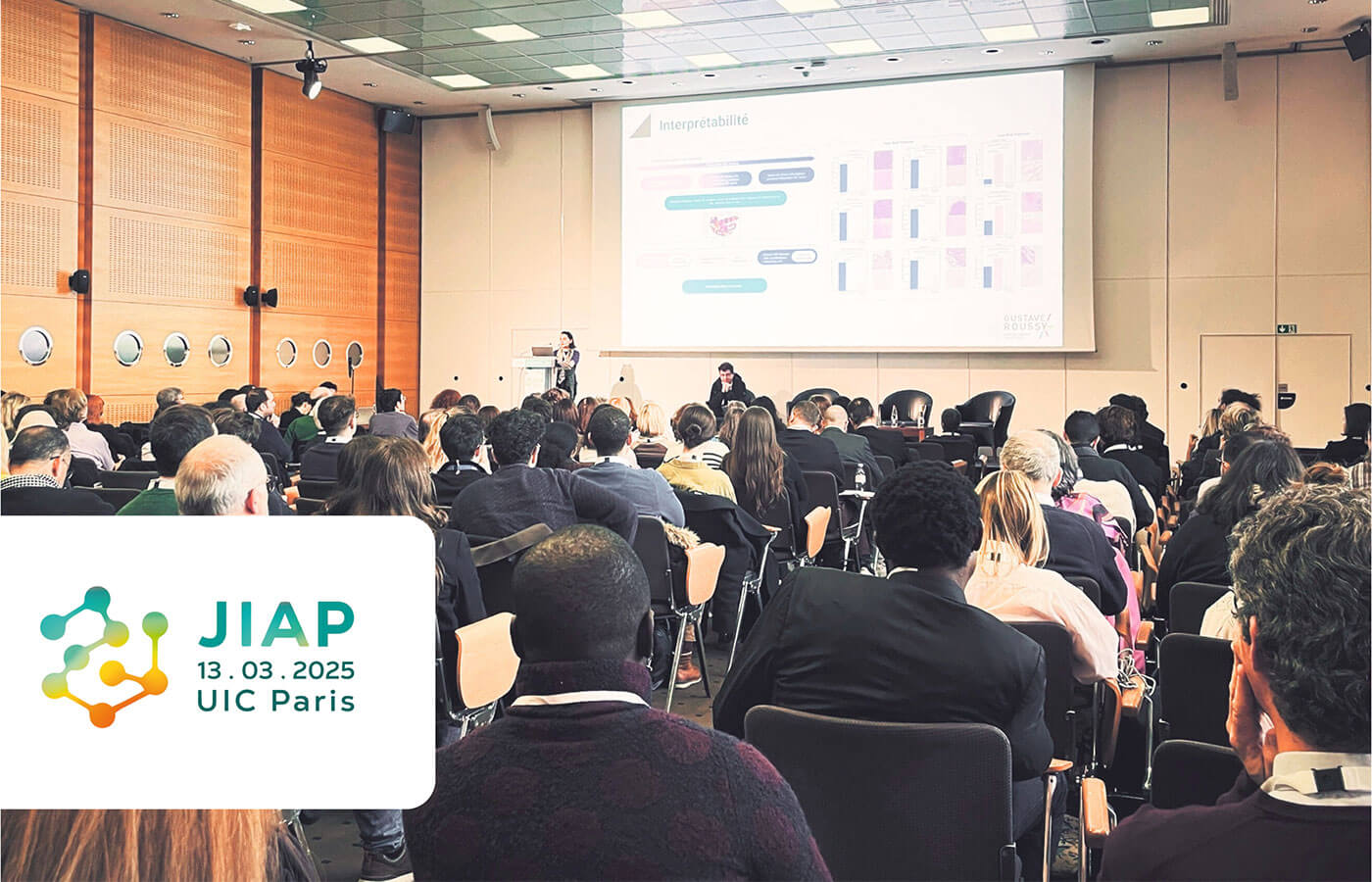
Key takeaways from JIAP 2025
The 2025 “1ère Journée IA et Pathologie” conference highlighted the transformative potential of AI in pathology while acknowledging current challenges and opportunities. This conference gave a comprehensive overview of the field's evolution from experimental applications to practical implementation considerations.
The program thoroughly covered numerous topics, ranging from AI fundamentals to concrete experience, including regulatory, technological, and ethical challenges.
Here are our key takeaways:
- Current AI implementation challenges: Despite a decade of development in medical imaging, AI tools still face reimbursement issues and integration challenges. Hence, the cost-effectiveness of AI tools in radiology remains a question. One of the concerns driving this is the "black box" nature of AI decision-making. That needs addressing through better explainability.
- Regulatory evolution: Important changes are coming with the European Health Data Space (EHDS) initiative and CNIL methodology updates. The EHDS framework specifically addresses data sharing mechanisms through designated intermediary organizations, but specific funding models are still under development and may vary by member state.
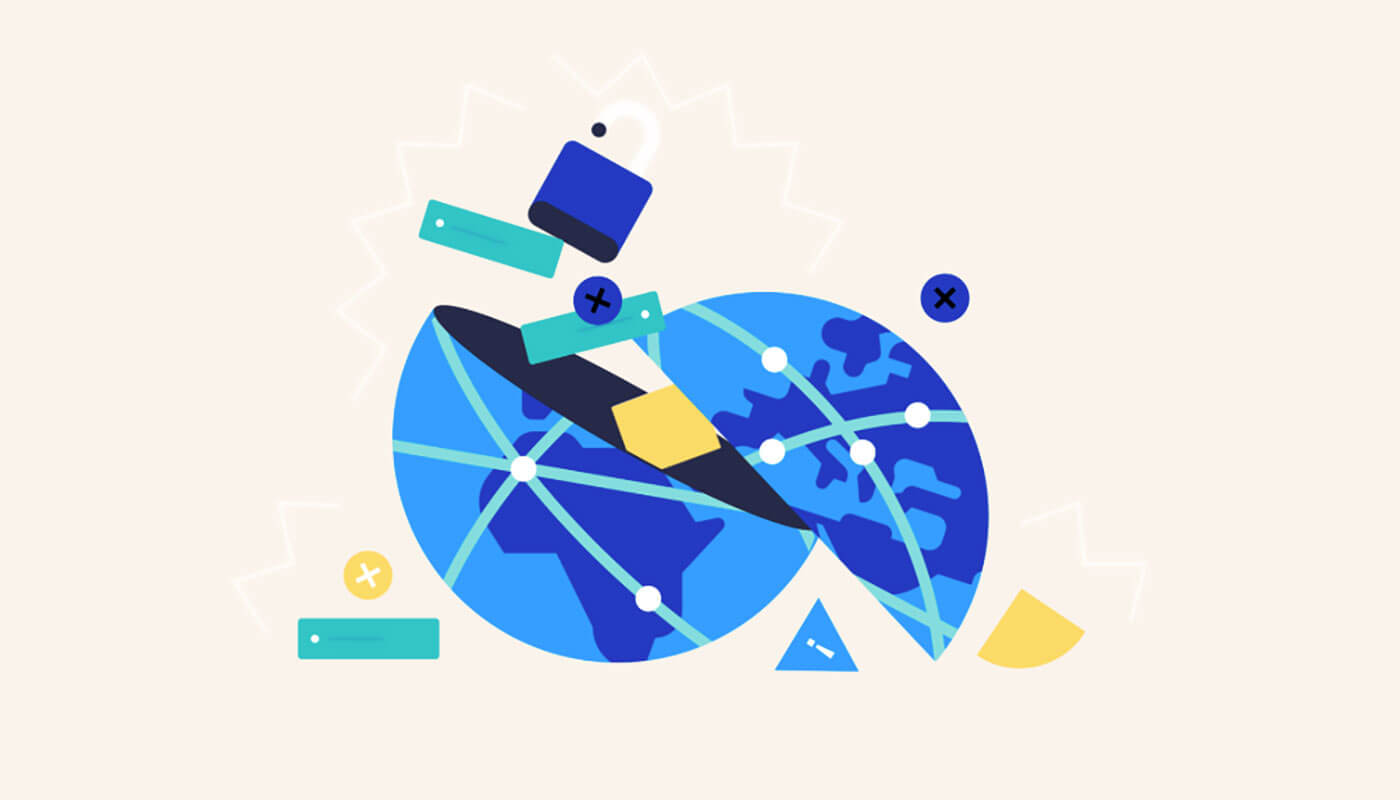
- Technical infrastructure: There is a critical need for standardized formats in pathology AI applications. Current challenges include managing multiple partial-task tools (current AI tools can only perform specific, limited tasks rather than complete end-to-end workflows) and ensuring seamless integration into existing systems.
- Validation framework development: The field is adopting robust validation methods including APPRAISE AI, QUADAS AI, and PROBAST+AI checklists, alongside EGAPP criteria for comprehensive evaluation of AI tools.
- Clinical implementation progress: Real-world applications are showing promise, as demonstrated by RlapsRisk® BC for breast cancer recurrence prediction. However, success requires realistic expectations and systematic validation approaches following the Gartner hype cycle model.

Conclusion
While AI in pathology shows immense promise, successful implementation requires a balanced approach considering technical, regulatory, and clinical aspects. The field is moving towards more standardized and validated solutions, with a clear focus on practical utility and patient benefit.
Unlock the "black box" by reading a comprehensive white paper on how AI models for pathology are developed here.